Category Archives: Research
Proceedings of the IEEE Best Paper Award: “Training Spiking Neural Networks Using Lessons from Deep Learning”
“Reducing Data Bottlenecks in Distributed, Heterogeneous Neural Networks” by Undergraduate Researcher Ruhai Lin Accepted at IEEE MCSoC-2024
“Evaluation and mitigation of cognitive biases in medical language models” published in npj Digital Medicine
Increasing interest in applying large language models (LLMs) to medicine is due in part to their impressive performance on medical exam questions. However, these exams do not capture the complexity of real patient–doctor interactions because of factors like patient compliance, experience, and cognitive bias. We hypothesized that LLMs would produce less accurate responses when faced with clinically biased questions as compared to unbiased ones. To test this, we developed the BiasMedQA dataset, which consists of 1273 USMLE questions modified to replicate common clinically relevant cognitive biases. We assessed six LLMs on BiasMedQA and found that GPT-4 stood out for its resilience to bias, in contrast to Llama 2 70B-chat and PMC Llama 13B, which showed large drops in performance. Additionally, we introduced three bias mitigation strategies, which improved but did not fully restore accuracy. Our findings highlight the need to improve LLMs’ robustness to cognitive biases, in order to achieve more reliable applications of LLMs in healthcare.
Link: https://www.nature.com/articles/s41746-024-01283-6
“Neuromorphic intermediate representation: a unified instruction set for interoperable brain-inspired computing” Published in Nature Communications
Spiking neural networks and neuromorphic hardware platforms that simulate neuronal dynamics are getting wide attention and are being applied to many relevant problems using Machine Learning. Despite a well-established mathematical foundation for neural dynamics, there exists numerous software and hardware solutions and stacks whose variability makes it difficult to reproduce findings. Here, we establish a common reference frame for computations in digital neuromorphic systems, titled Neuromorphic Intermediate Representation (NIR). NIR defines a set of computational and composable model primitives as hybrid systems combining continuous-time dynamics and discrete events. By abstracting away assumptions around discretization and hardware constraints, NIR faithfully captures the computational model, while bridging differences between the evaluated implementation and the underlying mathematical formalism. NIR supports an unprecedented number of neuromorphic systems, which we demonstrate by reproducing three spiking neural network models of different complexity across 7 neuromorphic simulators and 4 digital hardware platforms. NIR decouples the development of neuromorphic hardware and software, enabling interoperability between platforms and improving accessibility to multiple neuromorphic technologies. We believe that NIR is a key next step in brain-inspired hardware-software co-evolution, enabling research towards the implementation of energy efficient computational principles of nervous systems. NIR is available at neuroir.org
Link: https://www.nature.com/articles/s41467-024-52259-9
“Bridging the gap between artificial intelligence and natural intelligence” Published in Nature Computational Science
Link: https://www.nature.com/articles/s43588-024-00677-6
“SpikeGPT: Generative pre-trained language model with spiking neural networks” by Ph.D. Candidate Rui-Jie Zhu Published in Transactions on Machine Learning Research
New Preprint: “Scalable MatMul-free Language Modeling” by Ph.D. Candidate Ruijie Zhu
The cost of processing language models is insane. It is estimated that the computation demands of ChatGPT are >$100,000 p/day to serve billions of requests received.
Led by Rui-Jie Zhu, we have developed the first MatMul-free language model (VMM/MMM-free) to scale beyond billion-parameters. Our previous work with SpikeGPT tapped out at about 216M parameters, but our latest model has been able to go up to 2.7B parameters (only limited by compute). We’re pretty certain it can keep going.
We provide a GPU-optimized implementation that uses 61% less VRAM over an unoptimized implementation during training.
However, there are several operations in this model that GPUs aren’t yet fully optimized for, such as ternary operations. So Ethan Sifferman, Tyler Sheaves and Dustin R. built a custom FPGA implementation to really milk it, and we can reach human-reading throughput at 13W. A little less than the power consumed by the human brain.
Preprint: https://lnkd.in/gaWbg7ss
GitHub training code: https://lnkd.in/gKFzQs_z
Pre-trained models on HuggingFace: https://lnkd.in/gDXFjPdm
New Preprint: “Autonomous Driving with Spiking Neural Networks” by Ph.D. Candidate Ruijie Zhu
From the guy who built the first spiking language generation model, Rui-Jie Zhu has found a way to make spiking neural networks (SNNs) perform end-to-end autonomous vehicle control. This model takes a 6-camera input and integrates perception, prediction and planning together into a single model with approximately 75x less operations than ST-P3 at comparable performance.
Making SNNs push beyond toy datasets has been a tough time, but we’ve put a lot of effort into showing how to scale to challenging, real-world problems. The next step for this model is to push it into a closed-loop system. Deploying models like this on low-latency neuromorphic hardware can enable fast response times from sensor to control. This is necessary if we want to bridge the sim2real gap. I.e., by the time you take action, you don’t want your world to have changed by too much.
Rather than forcing “spiking” into applications for the sake of it, it’s important to take it to domains where there is a computational benefit – and I think this is one of them.
Preprint: https://arxiv.org/abs/2405.19687
“Knowledge Distillation Through Time for Future Event Prediction” Presented at ICLR by Undergraduate Researcher Skye Gunasekaran
Abstract: Is it possible to learn from the future? Here, we introduce knowledge distillation through time (KDTT). In traditional knowledge distillation (KD), a reliable teacher model is used to train an error-prone student model. The difference between the teacher and student is typically model capacity; the teacher is larger in architecture. In KDTT, the teacher and student models differ in their assigned tasks. The teacher model is tasked with detecting events in sequential data, a simple task compared to the student model, which is challenged with forecasting said events in the future. Through KDTT, the student can use the ’future’ logits from a teacher model to extract temporal uncertainty. We show the efficacy of KDTT on seizure prediction, where the student forecaster achieves a 20.0% average increase in the area under the curve of the receiver operating characteristic (AUC-ROC)
New Paper: “Optically Tunable Electrical Oscillations in Oxide-Based Memristors for Neuromorphic Computing” led by Collaborator Dr. Shimul K. Nath
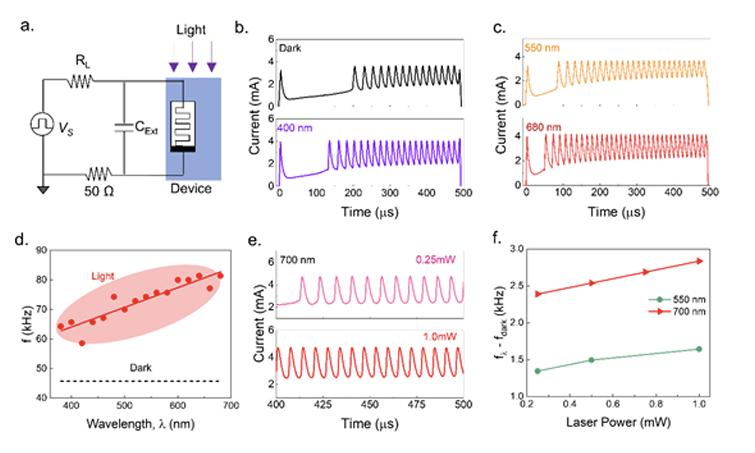
New Preprint: “Addressing cognitive bias in medical language models” led by Ph.D. Candidate Samuel Schmidgall
Preprint link here.
Abstract: The integration of large language models (LLMs) into the medical field has gained significant attention due to their promising accuracy in simulated clinical decision-making settings. However, clinical decision-making is more complex than simulations because physicians’ decisions are shaped by many factors, including the presence of cognitive bias. However, the degree to which LLMs are susceptible to the same cognitive biases that affect human clinicians remains unexplored. Our hypothesis posits that when LLMs are confronted with clinical questions containing cognitive biases, they will yield significantly less accurate responses compared to the same questions presented without such biases.In this study, we developed BiasMedQA, a novel benchmark for evaluating cognitive biases in LLMs applied to medical tasks. Using BiasMedQA we evaluated six LLMs, namely GPT-4, Mixtral-8x70B, GPT-3.5, PaLM-2, Llama 2 70B-chat, and the medically specialized PMC Llama 13B. We tested these models on 1,273 questions from the US Medical Licensing Exam (USMLE) Steps 1, 2, and 3, modified to replicate common clinically-relevant cognitive biases. Our analysis revealed varying effects for biases on these LLMs, with GPT-4 standing out for its resilience to bias, in contrast to Llama 2 70B-chat and PMC Llama 13B, which were disproportionately affected by cognitive bias. Our findings highlight the critical need for bias mitigation in the development of medical LLMs, pointing towards safer and more reliable applications in healthcare.
NSF MRSEC Seed Grant Awarded for the Co-Design of Next Generation Heterostructure-based Memristor for Neuromorphic Computing
New Paper: “To spike or not to spike: A digital hardware perspective on deep learning acceleration” led by Dr. Fabrizio Ottati in IEEE JETCAS
Find the paper on IEEE Xplore here.
Abstract:
New Paper: “Capturing the Pulse: A State-of-the-Art Review on Camera-Based Jugular Vein Assessment” led by Ph.D. Candidate Coen Arrow in Biomedical Optics Express
See the full paper here.
Abstract
Heart failure is associated with a rehospitalisation rate of up to 50% within six months. Elevated central venous pressure may serve as an early warning sign. While invasive procedures are used to measure central venous pressure for guiding treatment in hospital, this becomes impractical upon discharge. A non-invasive estimation technique exists, where the clinician visually inspects the pulsation of the jugular veins in the neck, but it is less reliable due to human limitations. Video and signal processing technologies may offer a high-fidelity alternative. This state-of-the-art review analyses existing literature on camera-based methods for jugular vein assessment. We summarize key design considerations and suggest avenues for future research. Our review highlights the neck as a rich imaging target beyond the jugular veins, capturing comprehensive cardiac signals, and outlines factors affecting signal quality and measurement accuracy. Addressing an often quoted limitation in the field, we also propose minimum reporting standards for future studies.
Brain-Inspired Machine Learning at UCSC: Class Tape-out Success
This quarter, I introduced Brain-Inspired Machine Learning as a course to University of California, Santa Cruz. And while machine learning is cool and all, it’s only as good as the hardware it runs on.
31 students & first time chip designers all took the lead on building DRC/LVS clean neuromorphic circuits. Students came from grad & undergrad backgrounds across various corners of the university. ECE, CSE, Math, Computational Media, Bioengineering, Psychology, etc. Many had never even taken an ECE 101 class, and started learning from scratch 2 weeks ago.
Their designs are now all being manufactured together in the Sky130 Process. Each design is compiled onto the same piece of silicon with TinyTapeout, thanks to Matt Venn and Uri Shaked.
We spent Friday night grinding in my lab while blaring metalcore tunes. All students managed to clear all checks. The final designs do a heap of cool things like accelerate sparse matrix-multiplies, event denoising, to simulating reservoir networks. I naturally had to squeeze in a Hodgkin-Huxley neuron in the 6 hours before the deadline (pictured).
Not sure if it’s the cost of living, or the mountain lions on campus, but damn. UCSC students have some serious grit.

New Paper: “Training spiking neural networks using lessons from deep learning” in the Proceedings of the IEEE
My baby was finally accepted for publication. Available open-access on IEEE Xplore.
Telluride Workshop: Open Source Neuromorphic Hardware, Software and Wetware
Prof. Jason Eshraghian & Dr. Peng Zhou were topic area leaders at the Telluride Neuromorphic Engineering & Cognition Workshop. Tasks addressed included:
-
porting open silicon (hardware) to neuromorphic engineering,
-
linking in-vitro neural networks (wetware) to neuromorphic computing, and
-
modelling and training those with spiking neural networks using neuromorphic software.
A project highlight includes the development of the Neuromorphic Intermediate Representation (NIR), an intermediate representation to translate various neuromorphic and physics-driven models that are based on continuous time ODEs into different formats. This makes it much easier to deploy models trained in one library to map to a large variety of backends.
Ruijie Zhu and Prof. Jason Eshraghian Present Invited Talk “Scaling up SNNs with SpikeGPT” at the Intel Neuromorphic Research Centre
Abstract: If we had a dollar for every time we heard “It will never scale!”, then neuromorphic engineers would be billionaires. This presentation will be centered on SpikeGPT, the first large-scale language model (LLM) using spiking neural nets (SNNs), and possibly the largest SNN that has been trained using error backpropagation.
The need for lightweight language models is more pressing than ever, especially now that we are becoming increasingly reliant on them from word processors and search engines, to code troubleshooting and academic grant writing. Our dependence on a single LLM means that every user is potentially pooling sensitive data into a singular database, which leads to significant security risks if breached.
SpikeGPT was built to move towards addressing the privacy and energy consumption challenges we presently run into using Transformer blocks. Our approach decomposes self-attention down into a recurrent form that is compatible with spiking neurons, along with dynamical weight matrices where the dynamics are learnable, rather than the parameters as with conventional deep learning.
We will provide an overview of what SpikeGPT does, how it works, and what it took to train it successfully. We will also provide a demo on how users can download pre-trained models available on HuggingFace so that listeners are able to experiment with them.
Link to the talk can be found here.
New Preprint: Brain-inspired learning in artificial neural networks: A Review led by Ph.D. Candidate Samuel Schmidgall
Abstract: Artificial neural networks (ANNs) have emerged as an essential tool in machine learning, achieving remarkable success across diverse domains, including image and speech generation, game playing, and robotics. However, there exist fundamental differences between ANNs’ operating mechanisms and those of the biological brain, particularly concerning learning processes. This paper presents a comprehensive review of current brain-inspired learning representations in artificial neural networks. We investigate the integration of more biologically plausible mechanisms, such as synaptic plasticity, to enhance these networks’ capabilities. Moreover, we delve into the potential advantages and challenges accompanying this approach. Ultimately, we pinpoint promising avenues for future research in this rapidly advancing field, which could bring us closer to understanding the essence of intelligence.
Link to the preprint here.
